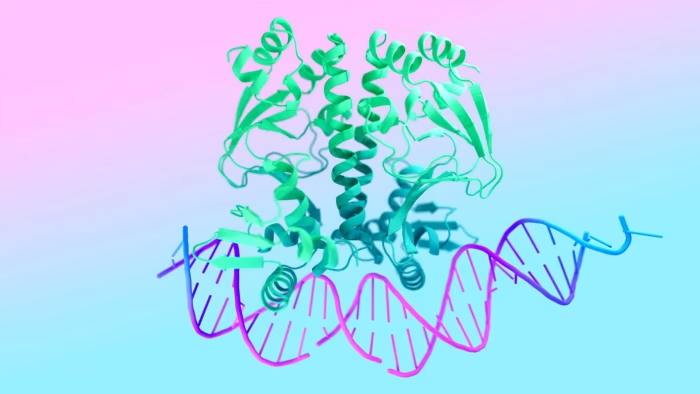
Unlocking Nature’s Secrets: AlphaFold 3 in Action
Unlocking Nature’s Secrets: AlphaFold 3 in Action
In a world where the mysteries of life’s building blocks are waiting to be unraveled, one groundbreaking technology is paving the way for scientific discovery like never before. Enter AlphaFold 3—a revolutionary leap in artificial intelligence that promises to transform our understanding of protein structures and their intricate roles in biology.
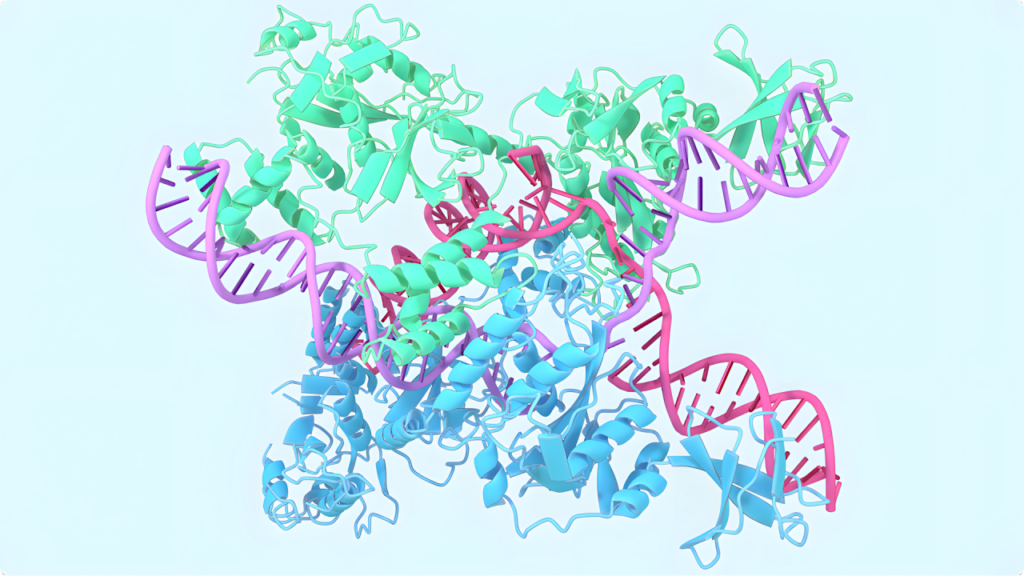
Imagine being able to predict how proteins fold with stunning accuracy, unlocking insights into diseases, drug development, and even environmental sustainability! Join us as we delve into this exciting frontier of science, exploring how AlphaFold 3 is not just decoding biological puzzles but is also shaping the future of research across disciplines. Get ready to witness nature’s secrets unfold before your eyes!
Introduction to AlphaFold 3 and its significance in the scientific community
The quest to understand protein folding has been a long and winding journey. For decades, scientists grappled with the complexity of how amino acid sequences fold into intricate three-dimensional structures.
Early attempts relied heavily on computational models that often fell short. The challenge was immense—thousands of proteins exist, each with unique configurations. Traditional methods were time-consuming and costly.
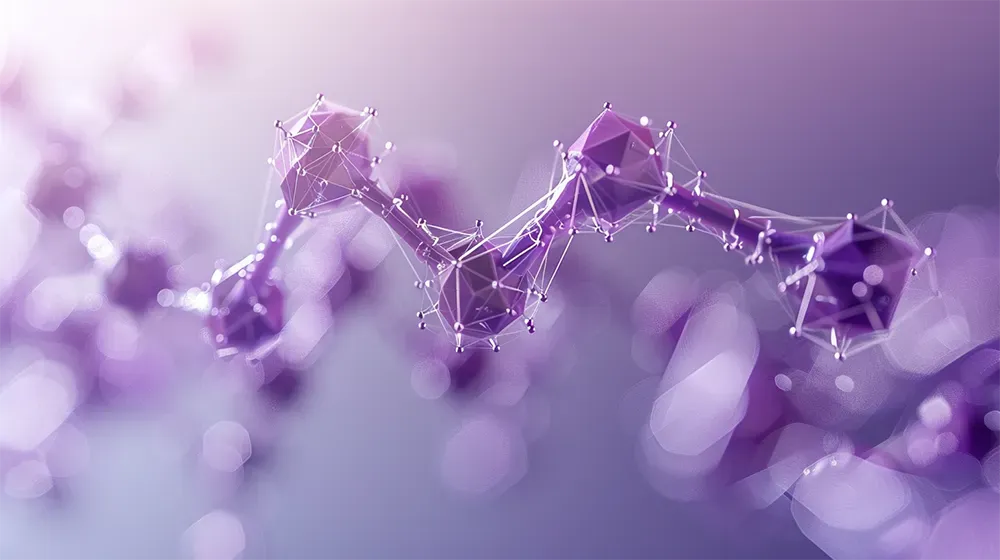
Then came breakthroughs from teams like Google’s DeepMind, which revolutionized the field. AlphaFold 1 made significant strides in accuracy but still faced limitations.
With the advent of AlphaFold 2, researchers witnessed an astonishing leap forward in predictive capabilities. It could predict folds at unprecedented levels of accuracy.
Now enters AlphaFold 3, building upon its predecessors while harnessing advanced neural networks and vast datasets. This innovation promises even more precise predictions and opens new avenues for scientific exploration across disciplines.
How does AlphaFold 3 work? Explaining the technology behind it.
AlphaFold 3 employs advanced deep-learning techniques to predict protein structures with remarkable accuracy. At its core, it leverages neural networks trained on a vast array of protein sequences and their corresponding 3D shapes.
The model processes the amino acid sequence of a protein, interpreting how these building blocks fold in space. It uses attention mechanisms similar to those found in natural language processing, allowing it to discern intricate patterns and relationships within proteins.
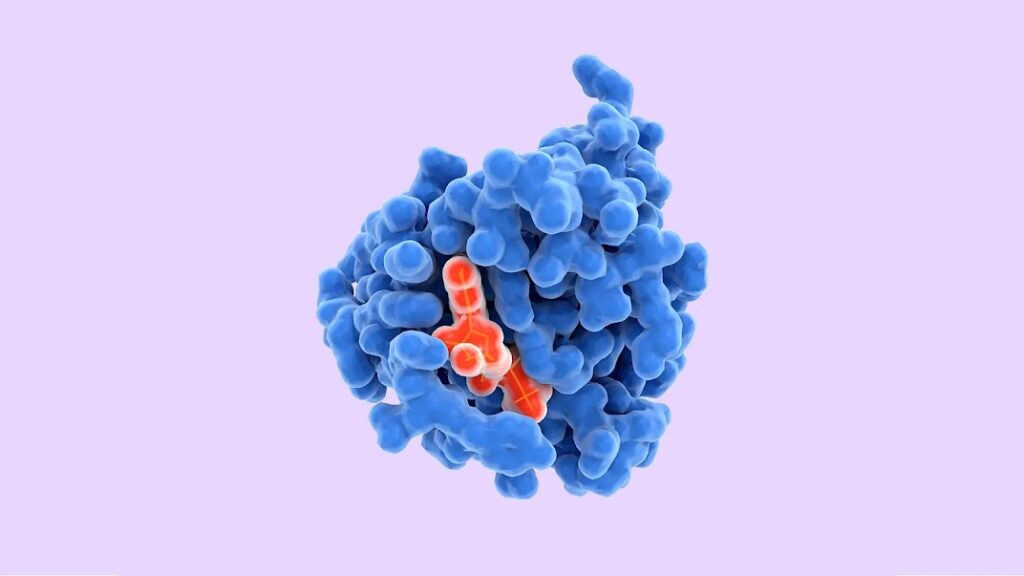
Additionally, AlphaFold 3 incorporates evolutionary data from multiple species. This insight enhances predictions by showing how certain folds have been conserved through evolution.
Ultimately, this sophisticated interplay between data and technology enables researchers to visualize complex biological structures more effectively than ever before. The implications for science are profound as the understanding of molecular biology advances rapidly due to such innovations.
Real-world applications of AlphaFold 3 in drug discovery, disease research, and more.
AlphaFold 3 is transforming the landscape of drug discovery. Predicting protein structures with remarkable accuracy enables scientists to identify potential drug targets faster than ever before.
In disease research, AlphaFold 3 plays a crucial role in understanding complex conditions like Alzheimer’s and cancer. Researchers can now visualize how proteins interact within cells, shedding light on molecular mechanisms behind these diseases.
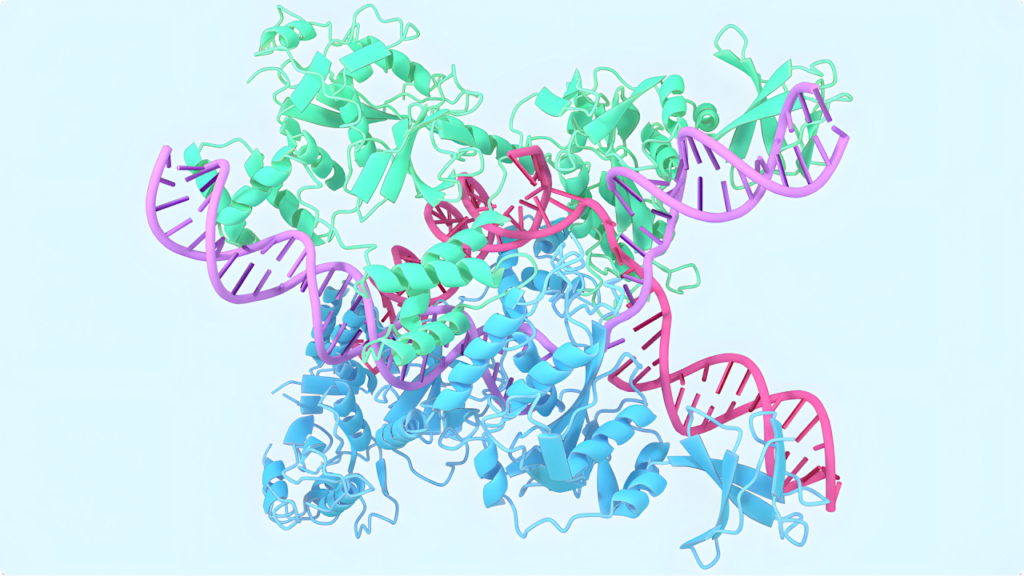
Additionally, its applications extend to synthetic biology and biotechnology. Engineers use AlphaFold 3 to design proteins for specific functions, paving the way for innovative therapies and sustainable solutions.
The technology’s rapid predictions save time and resources in lab settings. This efficiency accelerates advancements in medical science and increases the likelihood of successful treatments reaching patients sooner.
The impact of AlphaFold 3 on the future of science and medicine.
AlphaFold 3 is poised to revolutionize both science and medicine. Its ability to predict protein structures with remarkable accuracy opens new pathways for research.
Imagine a world where drug discovery accelerates significantly. Researchers can now design better therapies tailored to specific diseases. This technology could lead to breakthroughs in treating conditions previously deemed difficult, such as neurodegenerative disorders.
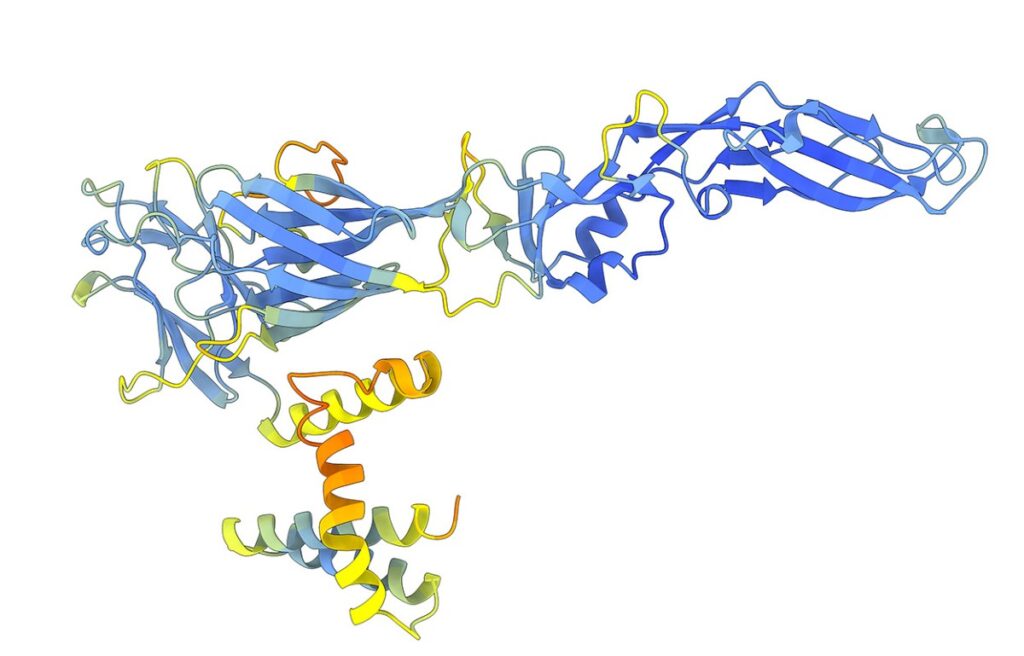
Moreover, understanding proteins at this level helps unravel complex biological processes. Scientists can explore how mutations affect function and contribute to various health issues.
The implications stretch beyond pharmaceuticals, too. Fields like biotechnology and agriculture stand to benefit from enhanced knowledge of molecular interactions, fostering innovation across the board.
As AlphaFold 3 continues evolving, it promises not just answers but new questions about life itself. The potential for groundbreaking discoveries remains vast and largely untapped.
Criticisms and concerns surrounding AlphaFold 3's accuracy and potential consequences.
Despite the excitement surrounding AlphaFold 3, it is not without its critics. Some researchers express concerns about the accuracy of its predictions. While many proteins are modeled effectively, there remain instances where results diverge from experimental data.
Another point of contention involves the implications for scientific research. Reliance on computational models might overshadow traditional lab experiments, leading to a potential decline in essential hands-on studies.
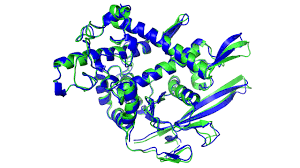
Additionally, ethical considerations come into play with regard to drug discovery. If incorrect predictions lead to flawed treatments, patients could face unforeseen risks.
The proprietary nature of DeepMind’s technology raises further issues around transparency and reproducibility in science. This lack of openness could hinder collaborative efforts that thrive on shared knowledge and methodologies within the research community.
Other similar technologies and their comparison to AlphaFold 3.
Several technologies aim to tackle the complex problem of protein folding. One prominent contender is Rosetta, a suite of software tools used for macromolecular modeling and design. While it has been around much longer than AlphaFold 3, it often requires extensive computational power and human expertise.
Another noteworthy technology is I-TASSER. This method relies on threading algorithms that align target sequences with known structures. Although effective in specific contexts, its accuracy can be inconsistent compared to AlphaFold’s advanced neural networks.
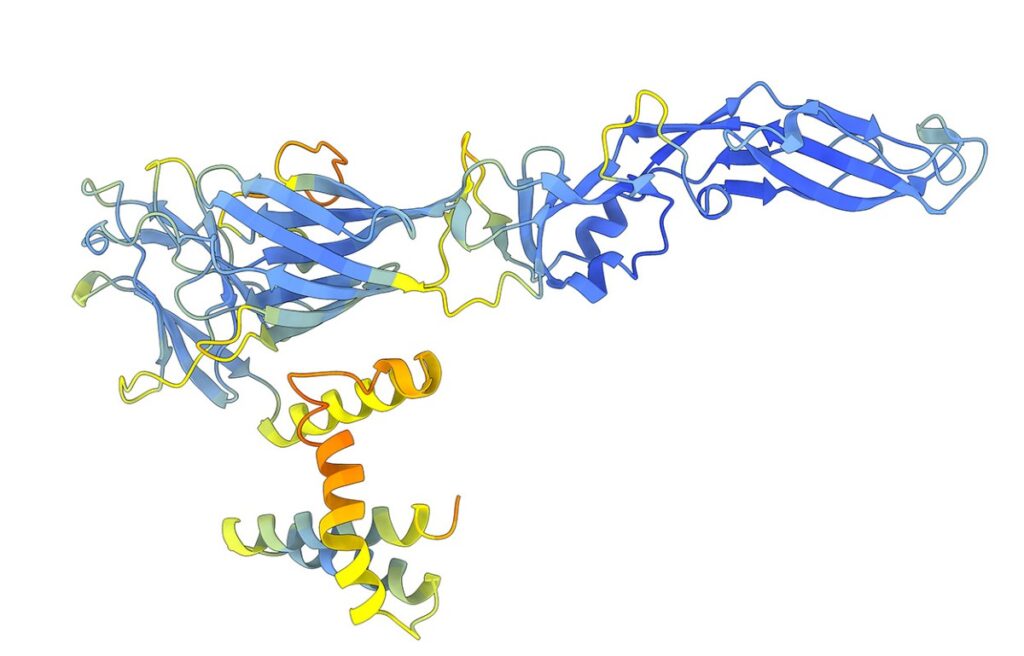
Then we have rosetta, which builds on deep learning principles but operates differently from AlphaFold 3 by focusing more on inter-residue contact predictions rather than overall structure generation.
Each approach brings unique strengths and weaknesses to the table, highlighting the ongoing evolution in our understanding of protein structures as scientists seek better solutions through competition and collaboration.
Conclusion: The potential of AlphaFold 3 in advancing our understanding of nature's secrets.
AlphaFold 3 represents a significant leap forward in our understanding of protein structure prediction. As Google DeepMind continues to push the boundaries of artificial intelligence, this technology is set to revolutionize not just biology but also medicine and pharmacology.
The ability to accurately predict how proteins fold opens up myriad possibilities for research. Scientists can now explore complex biological processes more efficiently. This advancement could lead to faster drug discovery, enabling researchers to identify potential treatments for diseases that have long eluded us.
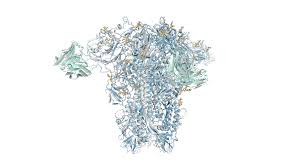
Moreover, as AlphaFold 3 enhances our grasp of molecular interactions and functions, it paves the way for breakthroughs in genetic engineering and synthetic biology. The implications extend beyond immediate scientific applications; they touch on ecological preservation and environmental challenges, too.
With its capacity to decode nature’s secrets at an unprecedented pace, AlphaFold 3 holds the promise of unraveling mysteries that have stumped humanity for centuries. This innovation invites scientists from various disciplines to collaborate and innovate like never before, fostering a spirit of exploration that resonates throughout the scientific community. Embracing such advancements will undoubtedly shape a future where understanding life at its most fundamental level becomes attainable—and perhaps even transformative—for all fields concerned with life sciences.